A paper and dataset describing an extensive 28 GHz mmWave measurement campaign to study outdoor-to-indoor signal propagation and user coverage has been accepted to appear at MobiHoc’22 [1]. The measurement campaign and analysis was a collaborative effort between Nokia Bell Labs, Universidad Tecnica Federico Santa Maria, Stuyvesant High School, the City College of New York (CCNY), and Columbia.
The campaign consisting of over 2,200 measurements was conducted in seven buildings around the West Harlem, NYC neighbourhood and Columbia campus and is mapped in the figure on the left below. The publicly available dataset provides the community with a unique set of mmWave measurements demonstrating the viability of indoors mmWave coverage at Hamilton Grange Middle School (HGMS), a public school located across from CCNY, within the COSMOS FCC Innovation Zone. The paper presents particularly good potential for over 2-gigabit indoor coverage at HGMS using mmWave base stations, and hope that the public dataset can be used in network planning and research to address the digital divide that often affects public schools and public housing in underprovisioned urban areas.
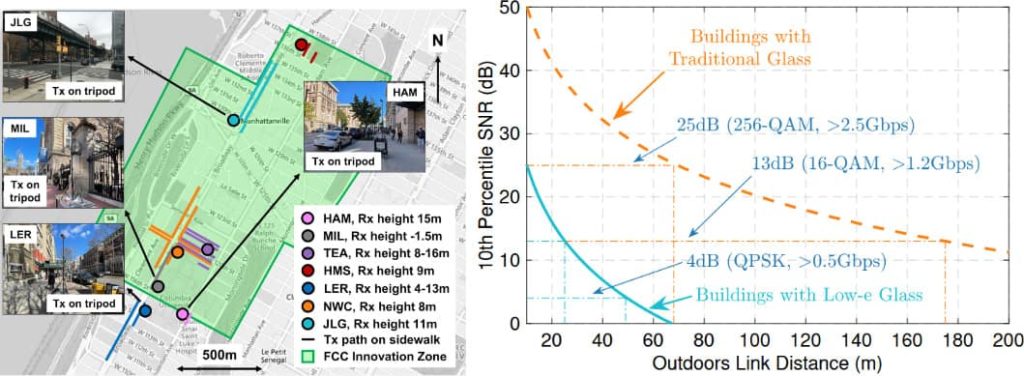
(Left) Map of measurement campaign. The channel sounder receiver (Rx) was placed in the seven marked buildings and the mmWave transmitter (Tx) moved along the marked paths, for a total of 40 scenarios. (Right) signal-to-noise ratio (SNR) against Tx-Rx distance for buildings with traditional glass (like HGMS) and buildings with Low-e glass, typically used in modern building construction. Traditional glass is significantly less lossy, leading to higher SNR and data rates in excess of 2.5 Gbps.
A paper on optical power spectrum prediction using machine learning in a multi-span ROADM system has been accepted to ECOC’22 [2]. This work was a collaborative effort between Duke University and Trinity College Dublin. This study separately characterized Lumentum ROADM-20 units deployed in the COSMOS testbed, each consisting of two erbium-doped fiber amplifiers (EDFAs): a booster and a preamp. For each EDFA in the multispan system, a comprehensive set of power spectrum measurements under diverse channel loading configurations were collected using the optical channel monitors (OCMs) that are built into the ROADM-20 units. Using the collected datasets, component-level EDFA gain spectrum models were developed using deep neural networks (DNNs), which predict the output power spectrum based on the channel loading configuration and input power spectrum. Transferring such individual models to a collective multi-span system, extensive experiments with diverse channel configurations show that the trained DNN-based EDFA model can accurately predict the power spectrum after 10 EDFAs with a mean absolute error (MAE) of 0.73 dB and 0.61 dB with two 5-span metroscale configurations, respectively.
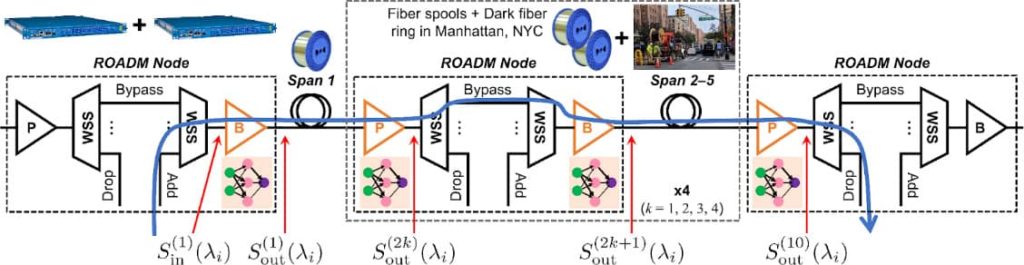
The experimental setup consisting of six ROADM nodes and five fiber spans in the COSMOS testbed, where the input is a flattened multi-channel signal source with varying channel configurations, and the power spectrum at the output of the each of the 10 EDFAs is predicted using the DNN model.
A paper on OpenRAN Gym, a new experimental toolbox for next-generation end-to-end Open Radio Access Network (ORAN) control systems, is available [3]. In order to test that control solutions developed using OpenRAN Gym are suitable for real-world use, they are tested on PAWR testbeds, including COSMOS. By making use of the USRP B210 radio nodes and bare metal servers available in COSMOS, the paper shows that the solutions developed in OpenRAN Gym for slicing and resource allocation are transferable to realistic network deployments. The same solution deployed on COSMOS shows a strong behavioural match to the solution deployed on the original lab-based testbed where it was developed.
References
[1] M. Kohli, A. Adhikari, G. Avci, S. Brent, J. Moser, S. Hossain, A. Dash, I. Kadota, R. Feick, D. Chizhik, J. Du, R. Valenzuela, and G. Zussman, “Outdoor-to-Indoor 28GHz Wireless Measurements in Manhattan: Path Loss, Location Impacts, and 90% Coverage,” in Proc. ACM MobiHoc’22, Oct. 2022. [download] [dataset (NIST)] [dataset (local)] [presentation]
[2] Z. Wang, E. Akinrintoyo, D. Kilper, and T. Chen, “Optical signal spectrum prediction using machine learning and in-line channel monitors in a multi-span ROADM system,” in Proc. ECOC’22, Sept. 2022. [download]
[3] L. Bonati, M. Polese, S. D’Oro, S. Basagni, and T. Melodia, “OpenRAN Gym: AI/ML Development, Data Collection, and Testing for O-RAN on PAWR Platforms”, arXiv:2207.12362 [cs.NI], July 2022. [download]